Meet our Speakers
Keynote Speakers
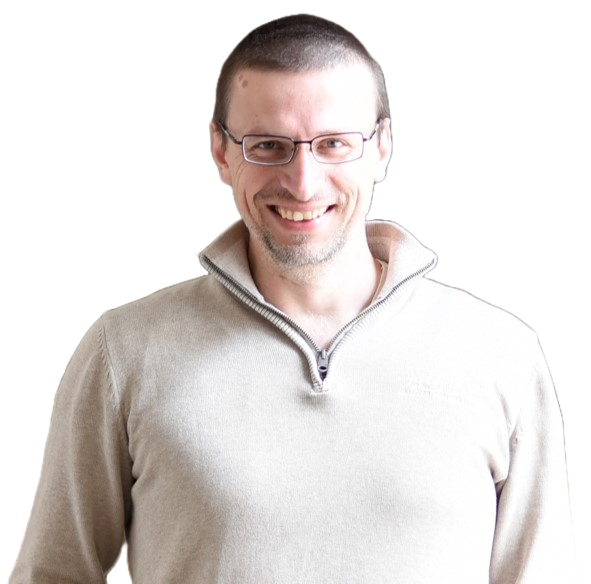
Marko Tkalčič
Associate Professor, Faculty of Mathematics, Natural Sciences and Information Technologies, University of Primorska, Koper, Slovenia
Personal WebsitePositive Psychology in User Modeling and Recommender Systems
Recommender systems, such as those used by Netflix, Spotify, and Amazon, rely on past user interaction data (e.g., clicks, views, purchases) to build models that predict the utility of items to recommend. Traditionally, these systems have been optimized to align with the commercial goals of the content providers—for instance, Netflix's recommendations are designed primarily to reduce subscription churn rather than to maximize user satisfaction. However, recent research has shifted focus towards enhancing the actual user experience. One promising direction explores this experience through the lens of positive psychology, particularly using the constructs of hedonic (pleasure-focused) and eudaimonic (purpose-driven) experiences. In this talk, I will introduce these concepts and demonstrate how they can be leveraged to model users and items, ultimately leading to more meaningful and satisfying recommendations.
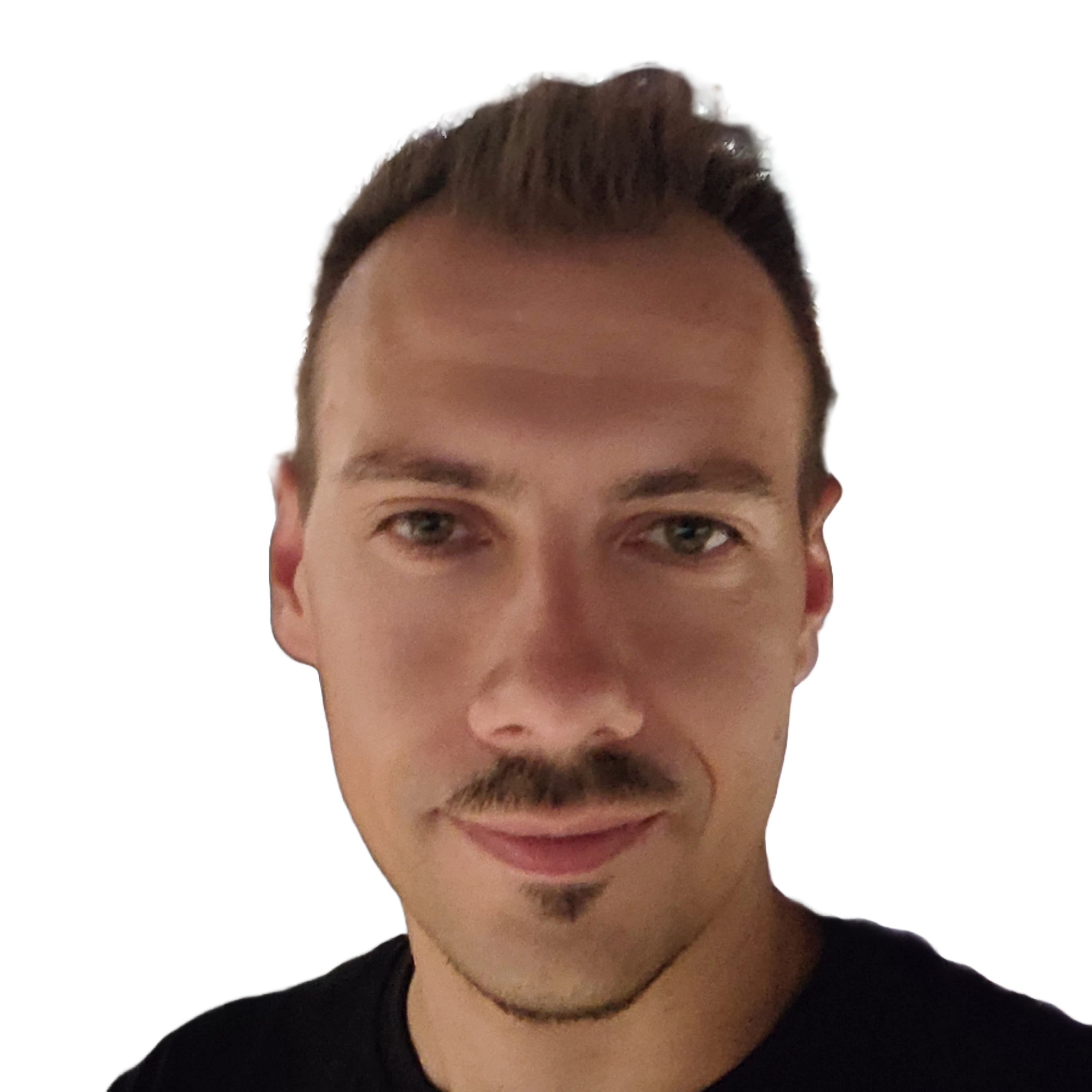
Marco Polignano
Professor Assistant, Computer Science Department, University of Bari Aldo Moro, Italy
Personal WebsiteLarge Language Models meets Human-Computer Interaction: The Large Agentic Models perspective
The advent of Large Language Models (LLMs) has revolutionized the field of Natural Language Processing, enabling machines to process, understand, and generate human-like language with unprecedented accuracy. As these models continue to advance, a new frontier is emerging at the intersection of LLMs and Human-Computer Interaction (HCI). This intersection, dubbed the "Large Agentic Models" (LAMs) perspective, holds immense potential for transforming the way humans interact with technology. In this perspective, LLMs are no longer merely linguistic processing engines, but rather agents that can perceive, reason, and respond to human inputs in a more intelligent and autonomous manner. This shift in perspective is driven by the increasing ability of LLMs to comprehend the nuances of human language, including context, intent, and sentiment and multimodal inputs to perform actions autonomously. As a result, the traditional boundaries between human and machine are blurring, and the future opportunities for more natural, intuitive, and effective human-computer interaction are unfolding. This position talk will touch this new frontiers of research stimulating discussion and novel research directions.
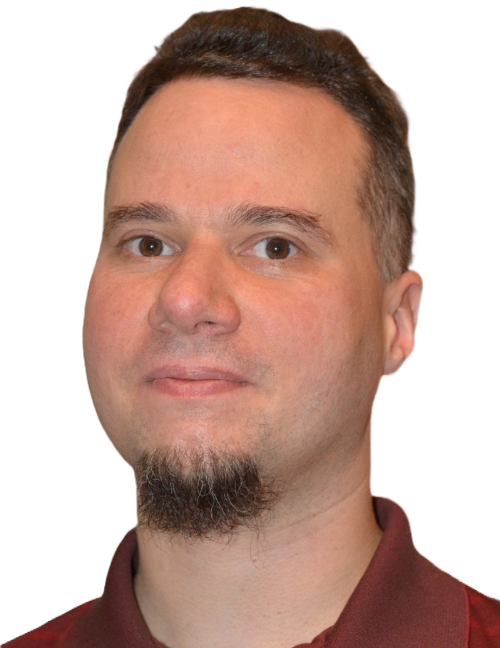
Title: Cognitive Biases in Human and Algorithmic Decision-Making Systems
Abstract: Cognitive biases have been extensively studied in psychology, sociology, and behavioral economics for decades. Traditionally, they were considered a negative human trait that leads to inferior decision making, reinforcement of stereotypes, or can be exploited to manipulate consumers, respectively. Lately, the AI research community has developed a growing interest into cognitive biases to better understand the influence of such biases in algorithmic decision-making systems, addressing classification, search, and especially recommendation tasks. We argue that cognitive biases manifest in different parts of the recommendation ecosystem and in various components of the recommendation pipeline, including input data (such as ratings or side information), recommendation algorithm or model (and consequently recommended items), and user interactions with the system. In my talk, I will introduce some established cognitive biases, such as conformity bias, homophily, and primacy/recency effects (position bias), but also some lesser-studied ones, including declinism, feature-positive effect, and IKEA effect. I will focus on their role pertaining to the two main entities involved in recommender systems - users and algorithms. Concretely, I will provide empirical evidence that feature-positive effect, IKEA effect, and cultural homophily can be observed in the context of recommender systems, and discuss their potential for exploitation. For this purpose, I will present several small experiments we conducted, in which we studied the pervasiveness of the aforementioned biases in the recruitment and entertainment domains. I will ultimately advocate for a prejudice-free consideration of cognitive biases to improve user and item models as well as recommendation algorithms.
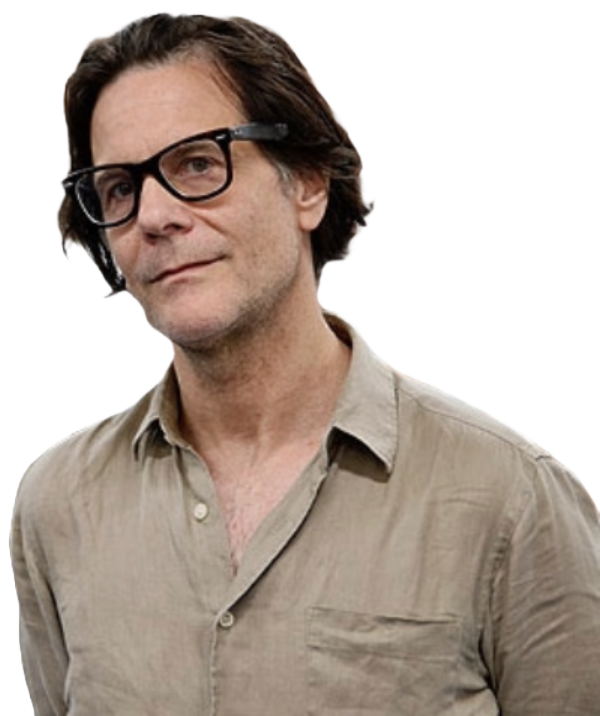
Title: Affect, Trust and Agency in Companion AI: From Synthetic Pets to Disembodied Lovers
Abstract: Until recently, the term ‘Personalized AI’ was associated with the ‘quantified self’ movement, yet smarter iterations have fuelled growing demand for a quantified ‘other’ now commonly referred to as Companion AIs. We argue that regardless of a Companion AI’s acumen for interpretating and simulating human emotions, a lack of user trust in AI can seriously obstruct its adoption. That trust is intertwined with machinic agency has implications for the ontological predilection of AI developers and humans to anthropomorize AI, especially, when the artifact takes on the status of an emotional surrogate masquerading as a carbon-based life form (Vanneste and Puranam, 2024). However, trust is highly subjective. The degree of trust we place in AI shapes our perception to its agency (Beerends and Aydin, 2024). AI Companions raise critical questions about human relations and interpersonal emotions, as well as ontological issues regarding the nature of love, intimacy and even ‘reality’ itself. Thus, there appears to be a need for research that encompasses non-anthropocentric views of consciousness, perception, self-awareness, cognition, agency, and importantly, multi-scalar potentialities of embodiment. Unlike other forms of AI, user trust in AI companions is constructed affectively through anthropomorphic promises and discursive practices of AI developers as well as the cognitive nonconscious (Hayles, 2017) interactions between user and their AI agent. I contend that like our relations with living persons and animals, normative understandings of trust in AI companions are based on authenticity, consistency, and intimacy (Gillath et al., 2021). I apply these parameters to my analysis through three distinct types of AI companions, specifically, artificial pets, friendship chatbots, and smart toys.
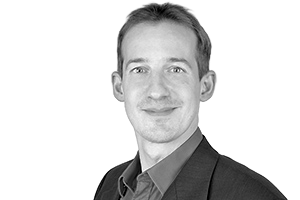
Title: Visualization for Explainability – From 2D Expert Tools to Intuitive Immersive Approaches
Abstract: Interactive visualizations are a powerful means of explainability for human beings. Often, explainable artificial intelligence (XAI) is equated to explainable machine learning (ML), encompassing ways of transforming black-box ML technologies into human-understandable glass-box solutions. AI, however, also encompasses symbolic approaches, for example, knowledge representations based on description logics. In addition, also formal methods are often centered around computational models with huge complexity that are hard to manage and comprehend, e.g., Markov decision processes. While both examples provide built-in explicability, results of reasoning processes or probabilistic model checking are complex, and experts need visual tools to support their work. The talk will highlight example solutions of interactive visualization tools for these areas that are the results of the Transregional Collaborative Research Center for Perspicuous Computing (CPEC). We will also reflect ideas of generalizing these specific solutions and making them more accessible for non-expert users. Thereby, immersive Mixed Reality visualizations can play a pivotal role and open up new ways to foster explainability for all.
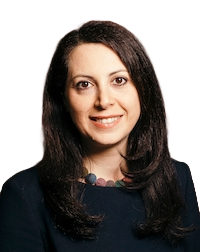
Sanaz Mostaghim
Full Professor of Computer Science, Otto von Guericke University of Magdeburg; Institute Director, Fraunhofer IVI; Member, Saxon Academy of Sciences
Personal WebsiteTitle: Human-Centric Multi-Objective Optimization and Decision-Making for Sustainable Mobility
Abstract: This talk will give an overview about the recent advances in decision-making techniques and their applications in transportation industry. Transportation plays more than ever a great role in our lives, while keeping us mobile, enabling fast and easy food and goods deliveries, and contributing to the global economy, its impact on climate change cannot be neglected. Therefore any improvement in optimization and decision-making in this field can have a considerable impact. This talk will provide insight into multi-objective optimization and decision-making algorithms for such applications and aims to provide future research directions. The incorporation of the users’ needs in the algorithms are discussed which supports the sustainability in the design of algorithms. The main focus is on the interplay between optimization and decision-making for human-centric decision support systems.
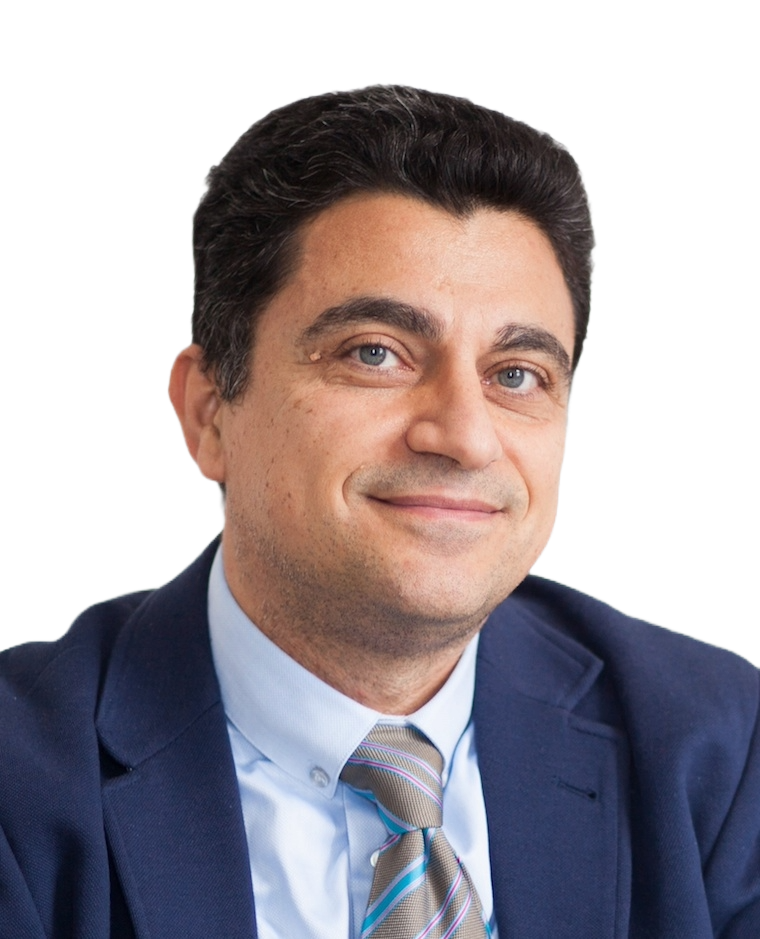
Carlo Sansone
Full Professor, Università degli Studi di Napoli Federico II, Dept. of Electrical Engineering and Information Technology, Naples, Italy
Personal WebsiteTitle: Human-Centred Artificial Intelligence Master (HCAIM): experiences and lessons learned
Abstract: This talk presents the Human-Centred AI Master's program, designed within the framework of the HCAIM project, funded by the European Community, which involved 4 universities, 3 research centres and 3 SMEs. Key experiences from the program's inception to its current state will be shared, highlighting interdisciplinary collaboration and student engagement strategies. In addition, lessons learned during the first two editions of the program will be discussed, offering valuable insights for future iterations and similar educational initiatives.